Сегментация дерматоскопических изображений новообразований кожи. Сравнение методик
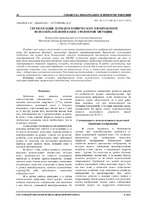
Date
2024Publisher
Another Title
Segmentation of dermatoscopic images of skin lesions. Comparison of methods
Bibliographic entry
Смалюк, А. Ф. Сегментация дерматоскопических изображений новообразований кожи. Сравнение методик = Segmentation of dermatoscopic images of skin lesions. Comparison of methods / А. Ф. Смалюк, М. С. Дешко, И. Д. Купчикова // Системный анализ и прикладная информатика. – 2024. – № 1. – С. 50-58.
Abstract
В работе рассмотрен ряд методик сегментации дерматоскопических изображений новообразований кожи для выявления областей, занимаемых данными новообразованиями. Выполнение сегментации необходимо как первый этап большинства методик компьютерной диагностики злокачественности новообразований. Ряд методик, таких как ABCDE, используют форму новообразования как один из критериев постановки диагноза, для других, таких как использование сверточных нейроных сетей, выделение новообразования позволяет повысить точность получаемых результатов. В работе рассмотрены три способа сегментации: пороговая обработка с использованием метода Оцу для вычисления величины порога, сверточная нейронная сеть, построеннная по архитектуре U-net, и аналогичная сверточная нейронная сеть с добавленным механизмом внимания. Рассмотрены достоинства и недостатки каждой из методик, а также возможности совместного их применения для получения наилучших результатов сегментации.
Abstract in another language
The work discusses a number of techniques for segmenting dermoscopic images of skin lesions to identify the areas occupied by these lesions. Segmentation is necessary as the first stage of most methods of computer diagnostics of malignancy of neoplasms. A number of techniques, such as ABCDE, use the shape of the tumor as one of the criteria for making a diagnosis; for others, such as the use of convolutional neural networks, identifying the tumor allows one to increase the accuracy of the results obtained. The work discusses three methods of segmentation: thresholding using Otsu's method to calculate the threshold value, a convolutional neural network built on the U-net architecture, and a similar convolutional neural network with an added attention mechanism. The advantages and disadvantages of each method are considered, as well as the possibility of using them together to obtain the best segmentation results. The paper considers the application of an algorithm based on a morphological projector for determining structural differences for comparing dermoscopic images. This will allow to identify changes that have occurred in skin lesions over time, for a more accurate diagnosis of their malignancy. The proposed algorithm makes it possible to detect differences in images even if there is a significant difference in the brightness and color levels of the compared images, and also ignores small insignificant details, such as noise, dermatoscope optics marks, hair, etc. A method for correcting the desynchronization of images using the structural similarity index as a similarity metric, and the sine-cosine algorithm as an optimization algorithm is proposed. The proposed algorithms were tested on dermatoscopic images and the possibility of their application was demonstrated.
View/Open
Collections
- № 1[8]